How to Beat the Odds
Using machine learning and computational techniques to predict a winning bracket
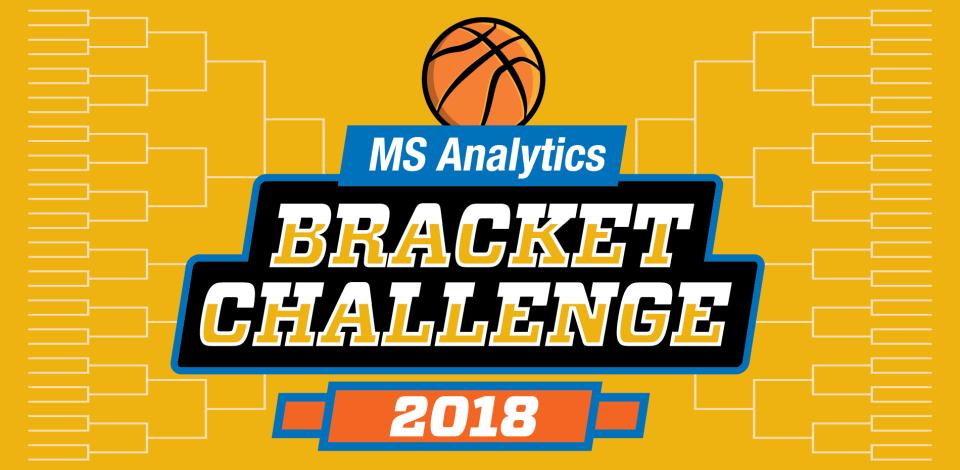
The National Collegiate Athletic Association (NCAA) Division I men’s basketball tournament is one of the most exciting parts of the year for United States sports fans, thanks in part to the annual contest to predict which teams will successfully progress through the tournament.
As one of the most wagered-upon sporting events in the U.S., an estimated 40 million Americans fill out a bracket each year to determine the ultimate champ. Busted brackets aren't uncommon due to upsets, human variability, and other unpredictable factors. In fact, the odds of randomly filling out a perfect bracket are about one in nine quintillions.
And that's where analytics comes in.
Joel Sokol, associate professor in Georgia Tech’s Stewart School of Industrial and Systems Engineering (ISyE) and director of the Online Master of Science in Analytics (OMS Analytics) degree program, turns his focus to college basketball every spring to beat the odds. He and Paul Kvam, an ISyE professor of statistics who was in the office next door, developed Georgia Tech’s Logistic Regression/Markov Chain (LRMC), a college basketball ranking system that has been making predictions for the last 15 years.
The LRMC uses basic scoreboard data to create a weekly ranking of all 68 teams during the tournament. The mathematical formula looks at every game and factors in the margin of victory, the winner or loser of each game, and game location.
“Since 2003, the LRMC has predicted about 74 percent of NCAA tournament games correctly, which is as much or more than all of the other methods we've tracked over the years," said Sokol. "Tests show that LRMC predicts outcomes better than expert polls, formulas, other computer models, and even the tournament seeds themselves."
Machine-learning research such as this has brought a powerful approach to solve problems and make decisions and goes hand-in-hand with the OMS Analytics program curriculum.
“The models and methods we teach here can be incredibly powerful for understanding systems, making predictions, and recommending decisions for a huge range of businesses and industries," remarked Sokol.
Inspired by this year's tournament and the success of LRMC, the OMS Analytics program launched an inaugural MS Analytics Bracket Challenge. Participants were given a virtual hand from Sokol to use LRMC — either to delve deep into each match up or just to fill out a competitive bracket. Over 130 students participated in the challenge, and although no one had a perfect bracket, the ultimate bragging rights went to future OMS Analytics student and MS Analytics Bracket Challenge winner, Sean Dolan.
As another college basketball season has come to an end, Sokol looks forward to the continuation of the LRMC legacy and challenging future participants to create and use their own predictive models not only to predict the perfect bracket but also to solve real-world problems.
Interested in learning more about LRMC? Sokol breaks how we utilize its data in the video below.