Practical Data Science and Machine Learning for Engineers
- Overview
- Course Content
- Requirements & Materials
COURSE ID: ELEC 1005P
Practical Data Science and Machine Learning for Engineers
Course Description
With the growing importance of data and data processing across all industries, it is critical for modern engineers to be nimble data scientists. For engineers who are not professional software developers, it can be tricky to break into the ecosystem of modern tooling that is required to efficiently process and learn from data. The focus of this course is to introduce the tools, theory, and methods for working with applied data science and machine learning (DS/ML). You will learn how to use and interact with open source DS/ML tools, the theory behind canonical ML algorithms, and practical methods and workflows for learning from data. This class is project based, and you will be guided through a series of practical data science problems. In addition, you will learn the DevOps skills required to be productive as a DS/ML engineer.
DEVOPS FOR DATA SCIENCE
- Efficiently integrate with Google, Amazon, and Azure Cloud products to create remote and repeatable computing environments
- Linux command line fundamentals for installing and maintaining production enterprise DS/ML pipelines
DATA SCIENCE BASICS IN PYTHON
- How to install Jupyter and how to use it on remote machines
- Deep dive into data manipulation with Pandas and Numpy
- Data visualization theory and practice using Matplotlib and Seaborn
MACHINE LEARNING THEORY
- Introduction and review of ML theory and algorithms
- Supervised learning, unsupervised learning, ensemble methods, boosting, deep neural networks
MACHINE LEARNING PRACTICE
- Classical ML techniques and conventions using Scikit-Learn
- Deep neural network learning using Tensorflow and Keras
SCALING DATA PIPELINES
- Processing big data with Hadoop and PySpark
- Creating repeatable production batch job pipelines using Luigi
Materials
Required - Student Must Provide
- Laptop that can access the internet over WiFi. All examples and coding will be run in the cloud, so the only software required is the Chrome Web Browser.
Who Should Attend
This course is designed for engineers, scientists, and managers from commercial industry, educational institutions, and government agencies. A core toolset will be covered that is relevant to skills across almost any modern industry, from science to advertising to industrial automation.
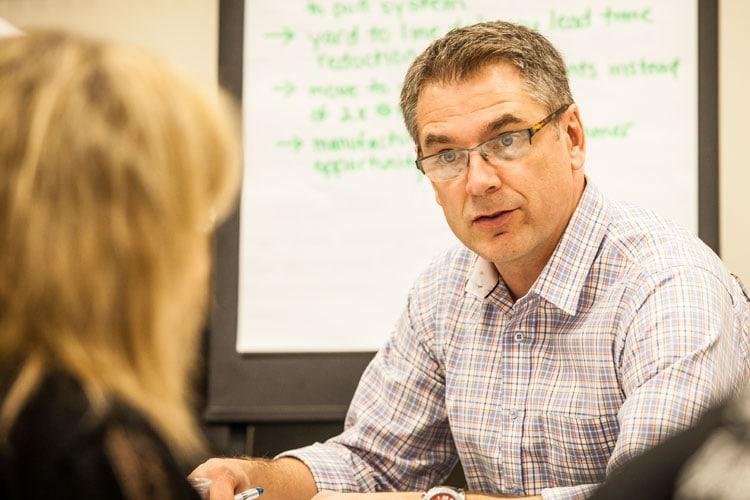
What You Will Learn
- Modern developer tools
- Data science workflow
- Data science with Python in a browser
- Manipulating data in Python
- Visualization stories with data
- Machine learning theory
- Machine learning workflow and pipelines
- How to deal with bigger data
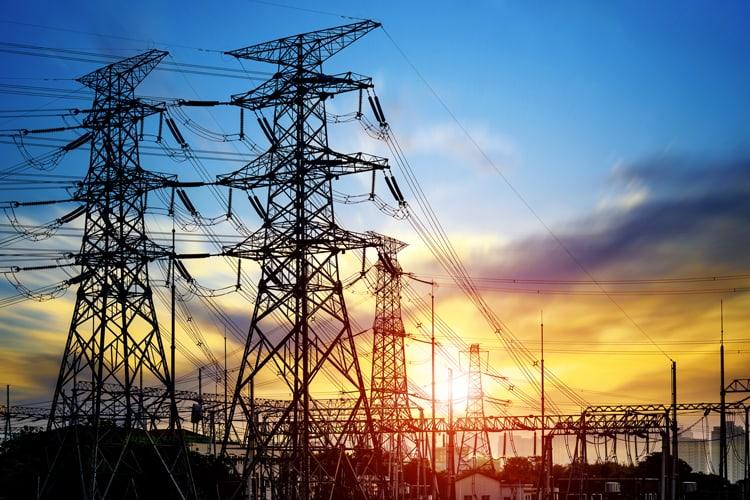
How You Will Benefit
- Implement fully functioning machine learning pipelines.
- Apply modern DS/ML toolchain to practical problems.
- Become a proficient user of Python and Jupyter.
- Implement data visualization for effective data storytelling.
- Apply big data tools, such as Hadoop, Spark, and Amazon Web Services to data problems.
-
Taught by Experts in the Field
-
Grow Your Professional Network
The course schedule was well-structured with a mix of lectures, class discussions, and hands-on exercises led by knowledgeable and engaging instructors.
Related Programs
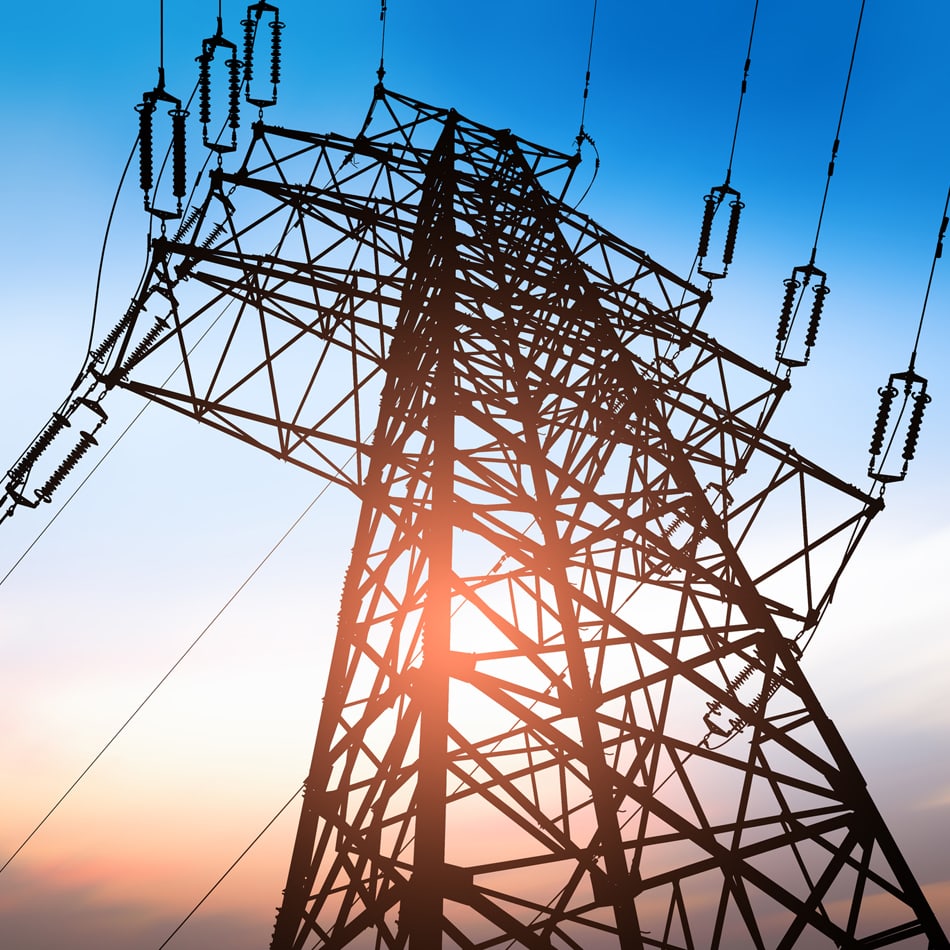
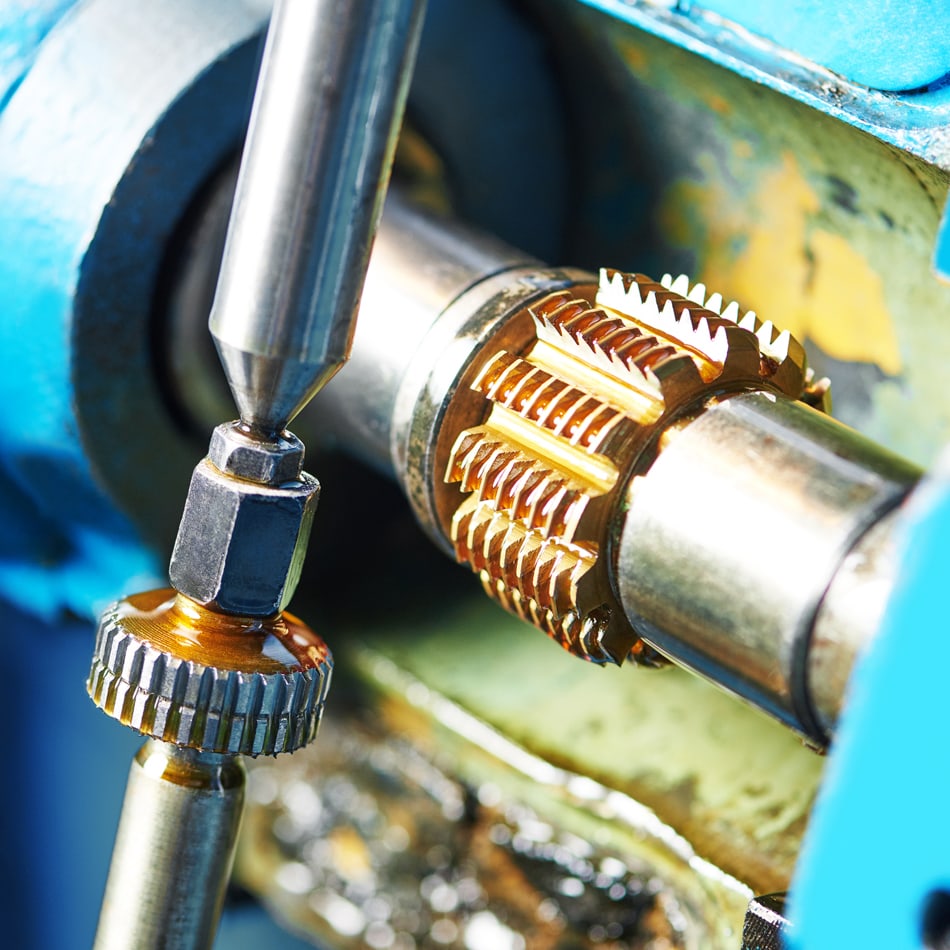
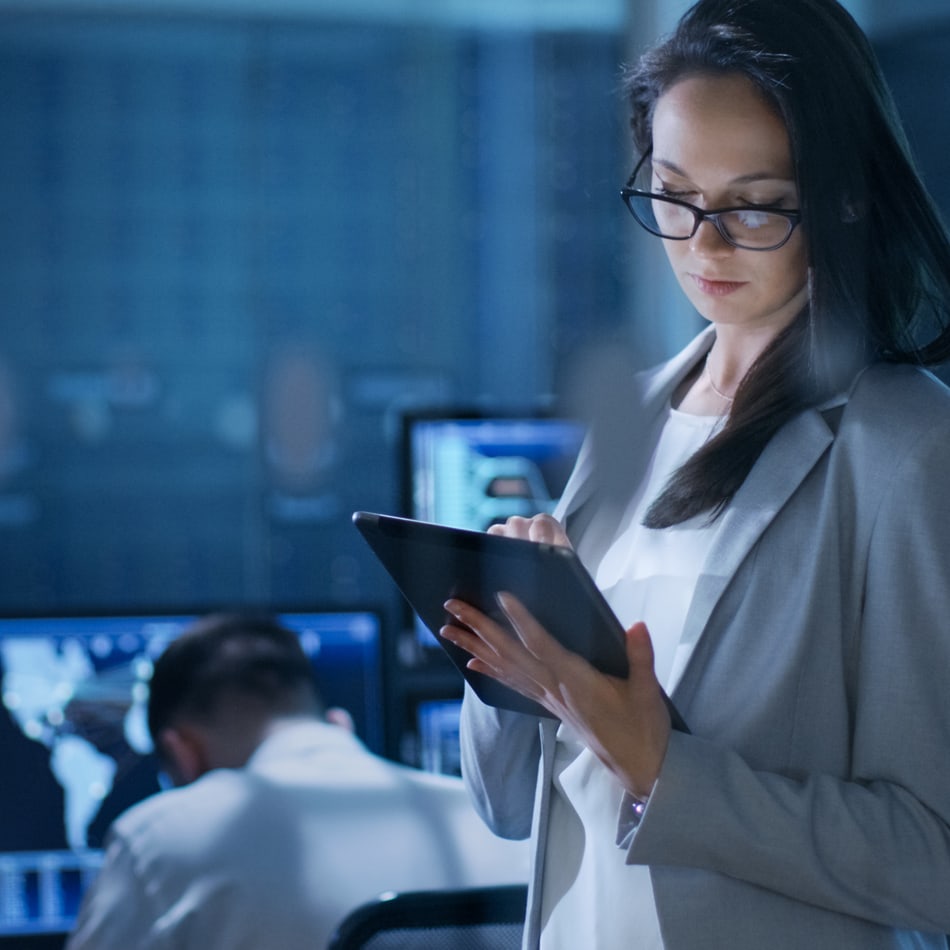
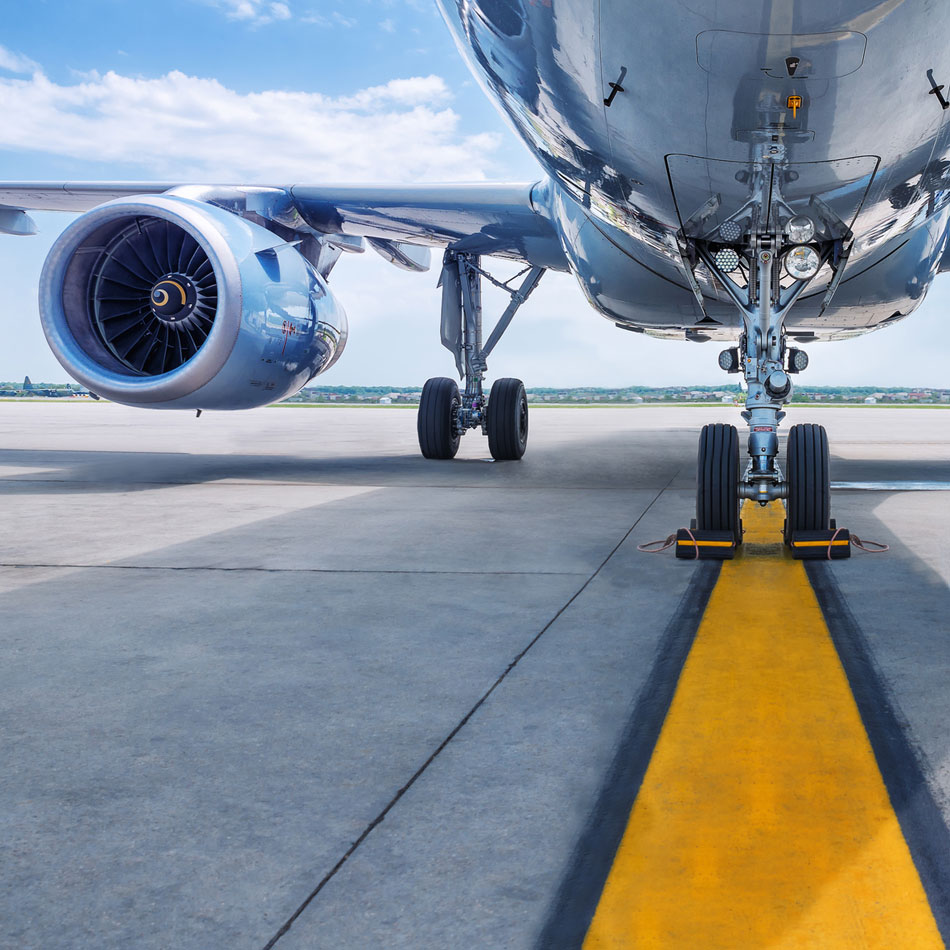
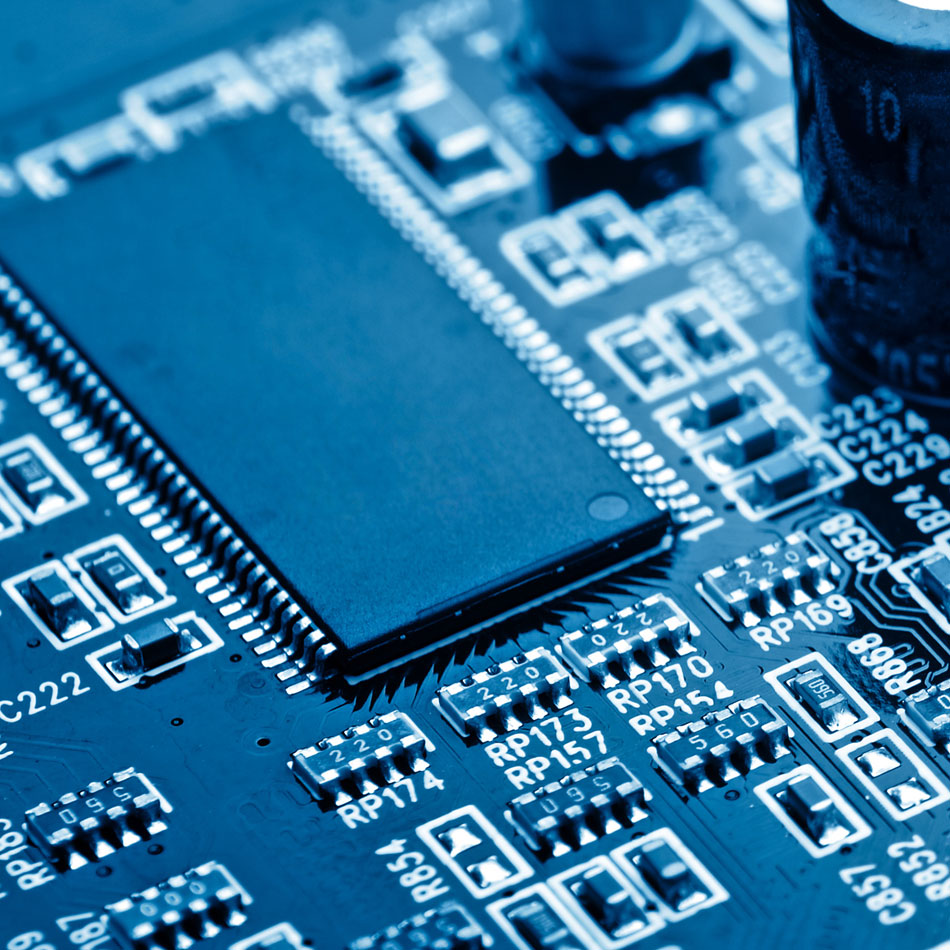
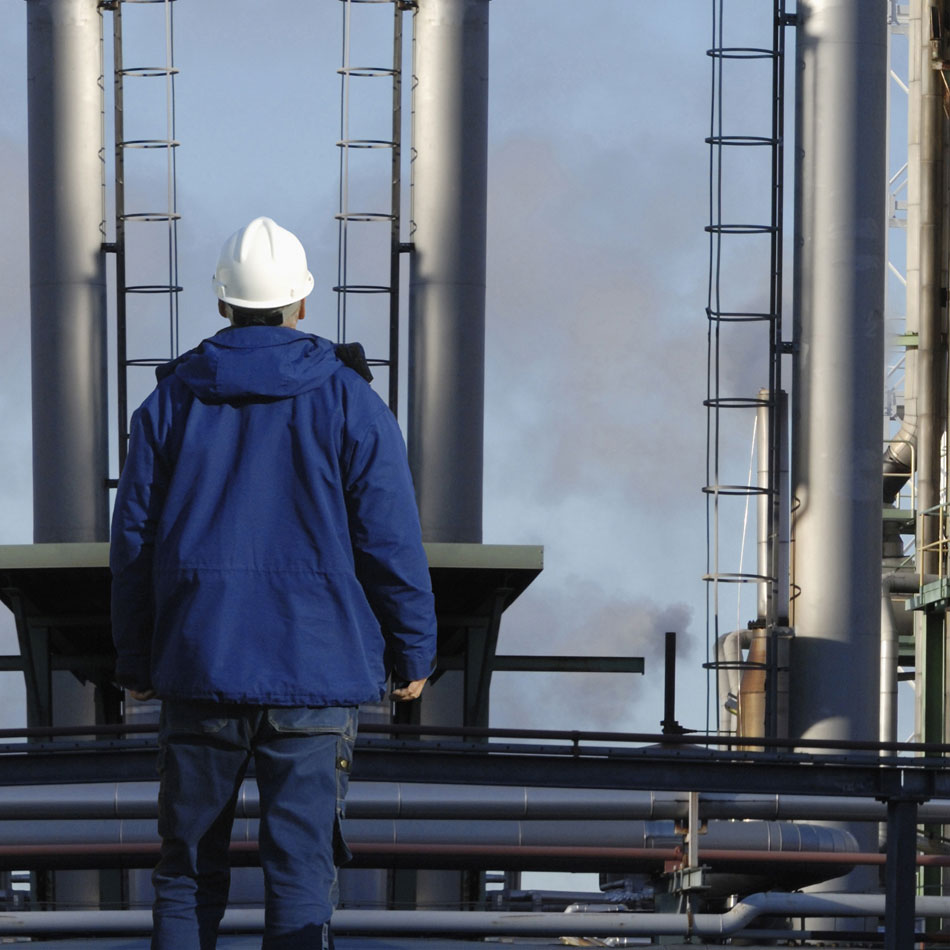
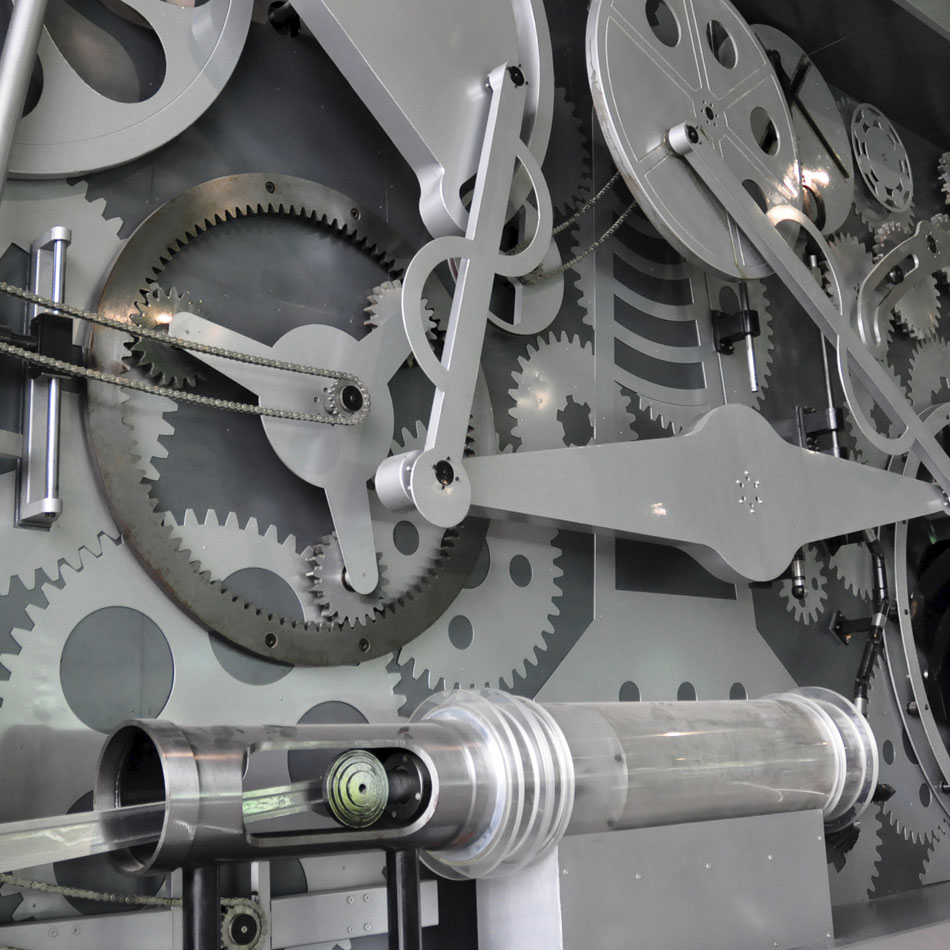
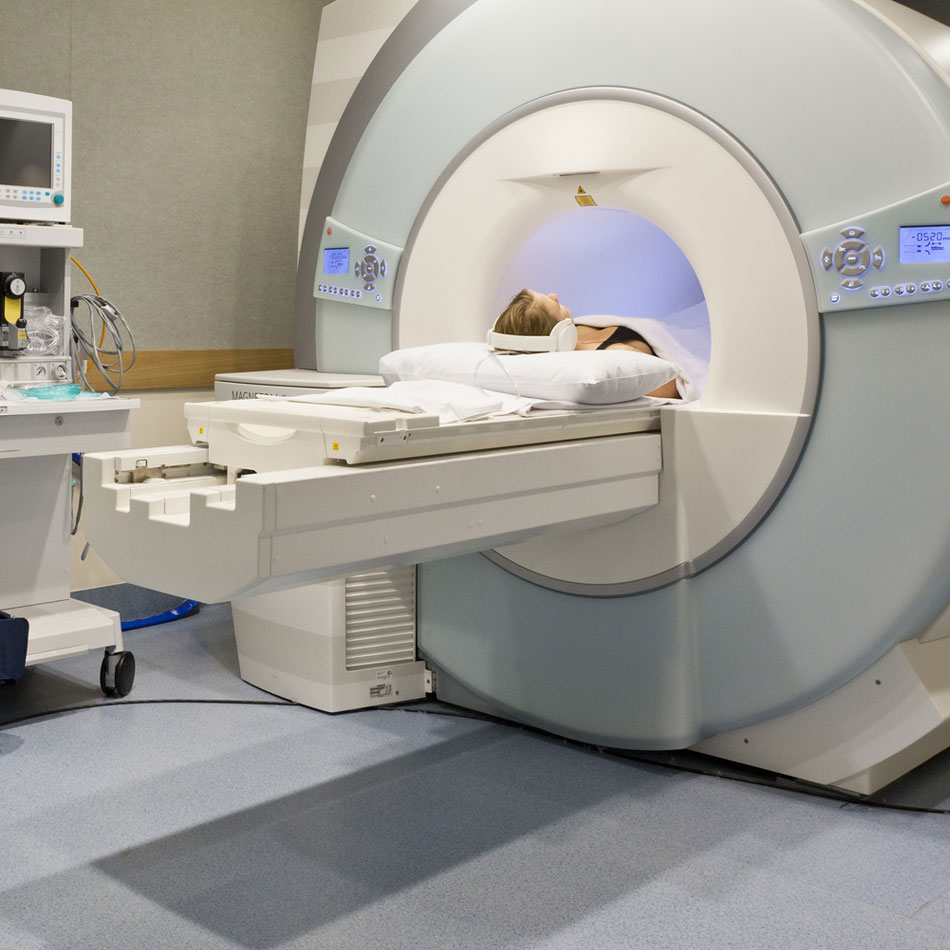
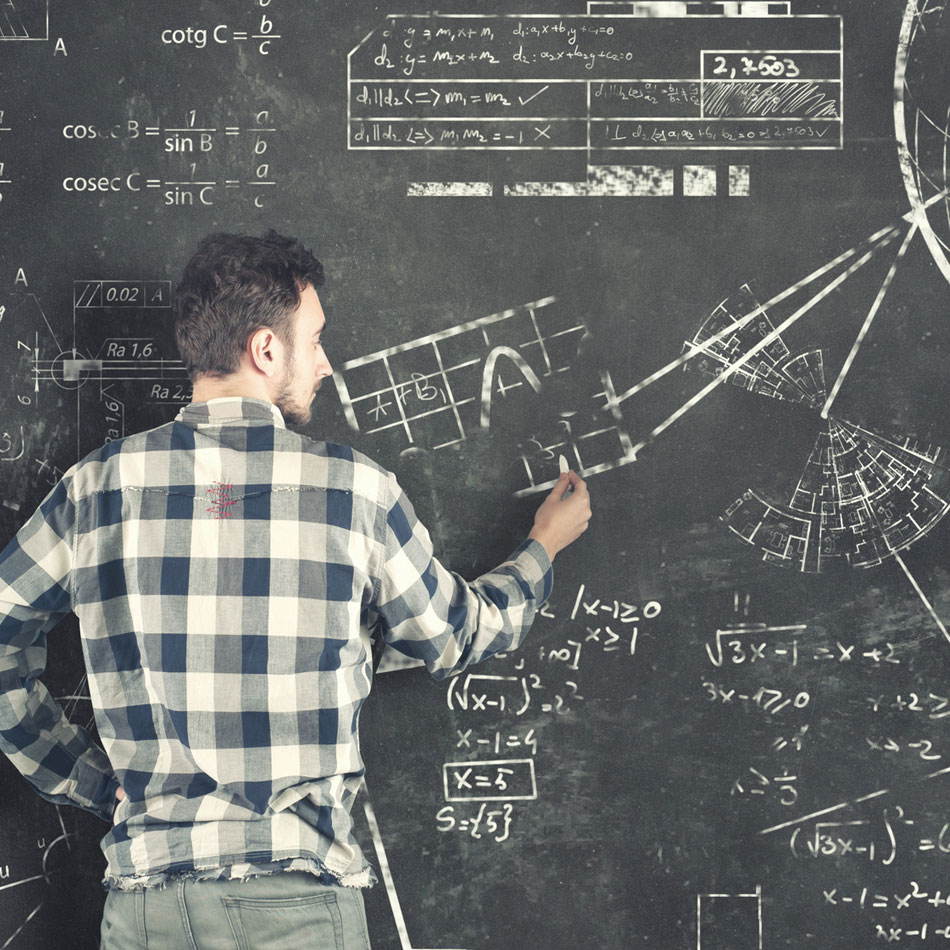
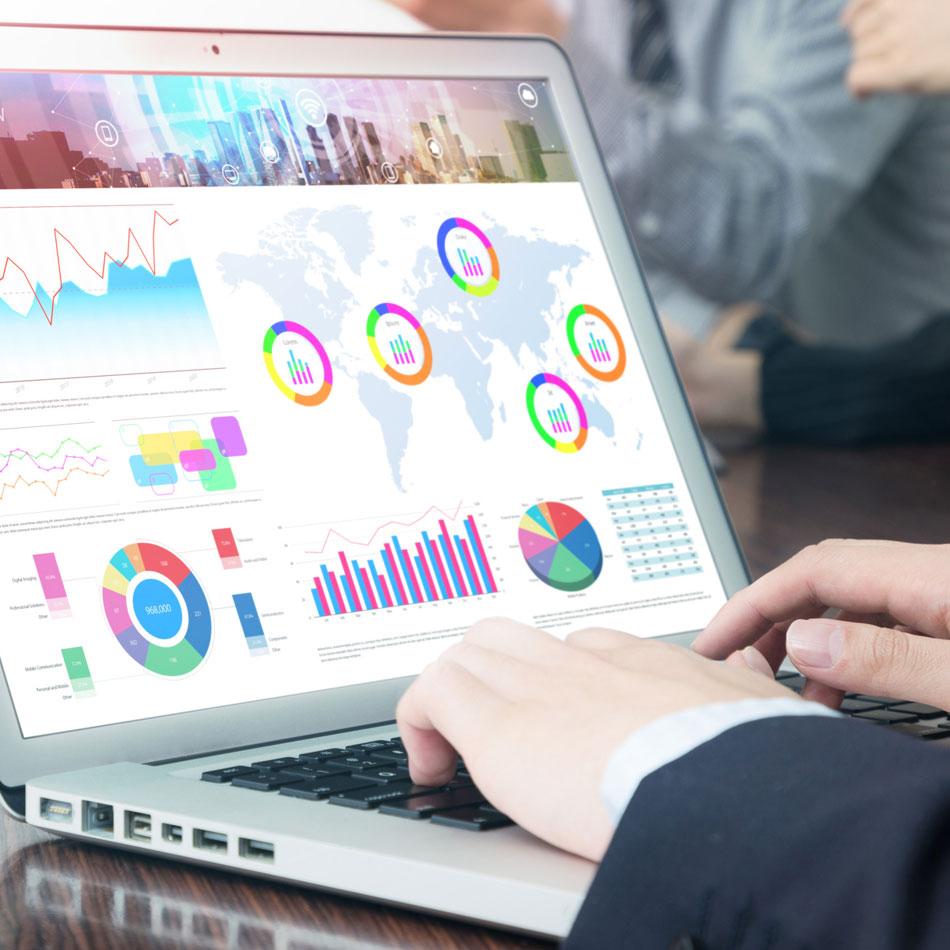
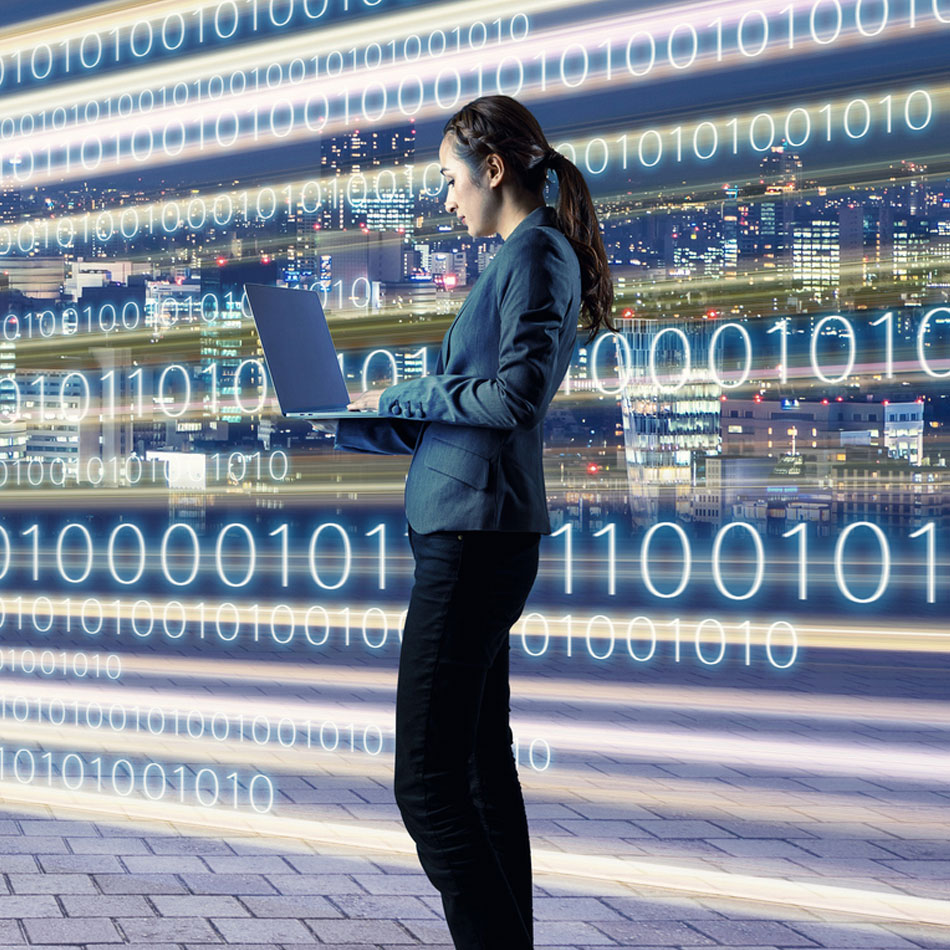
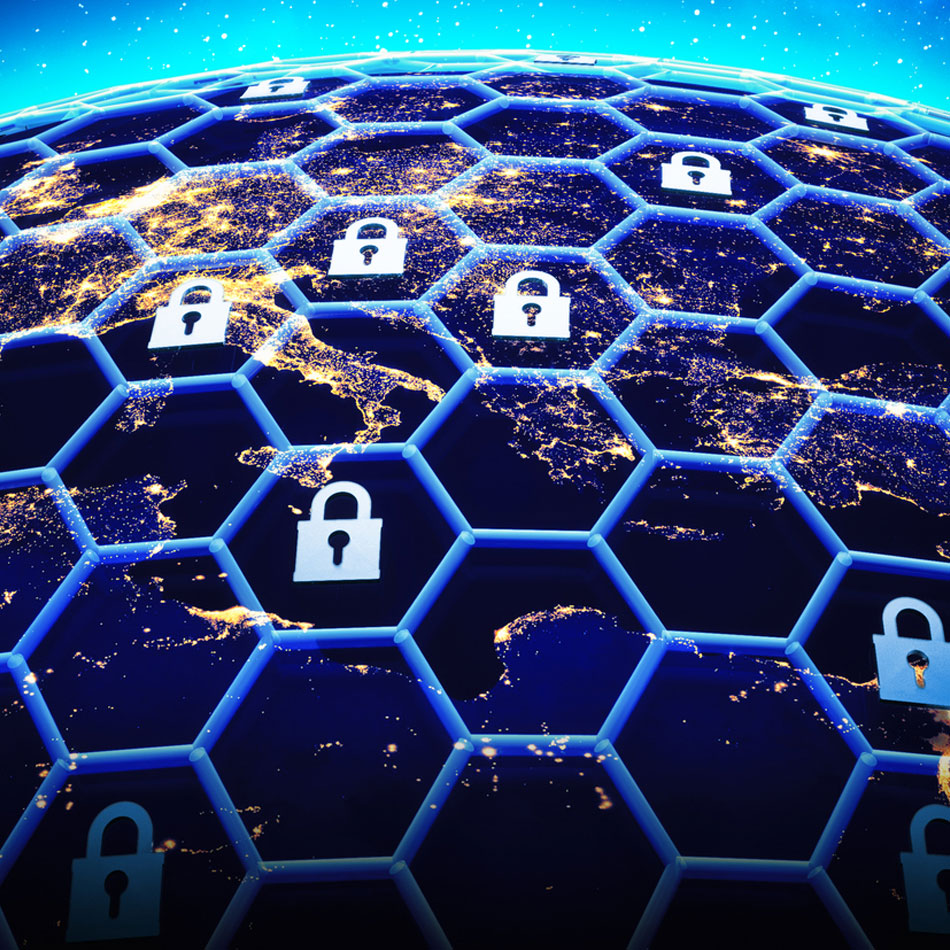
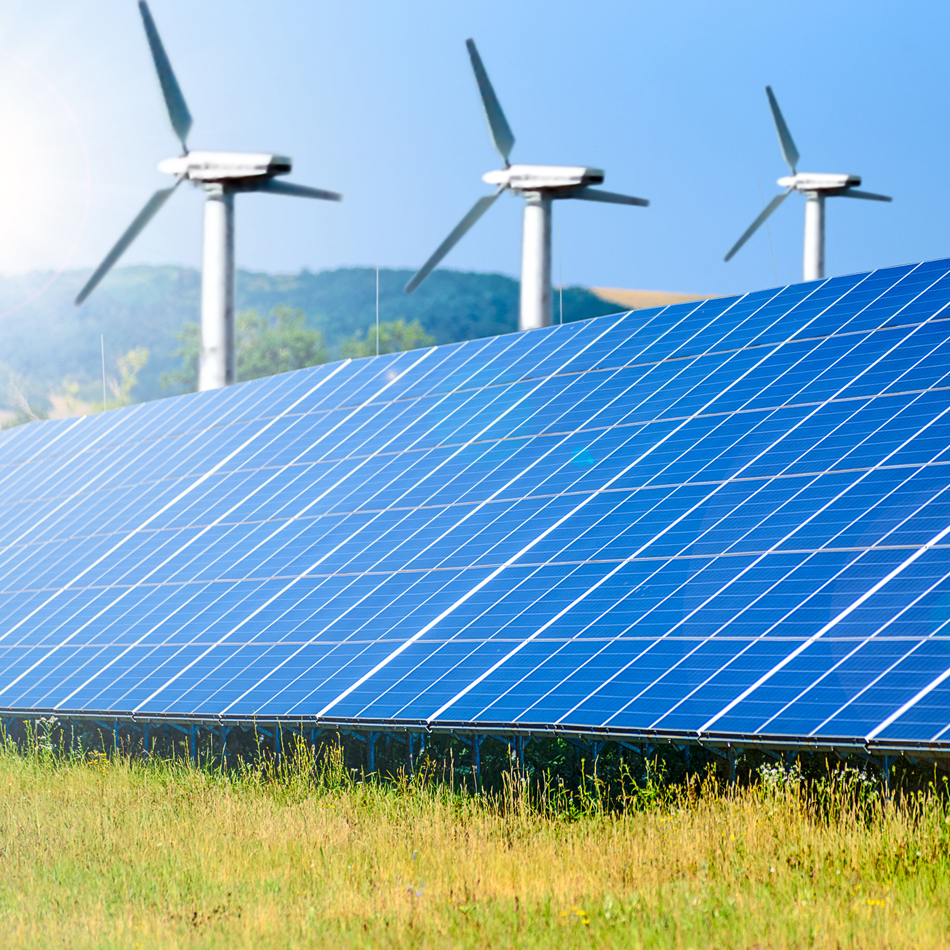
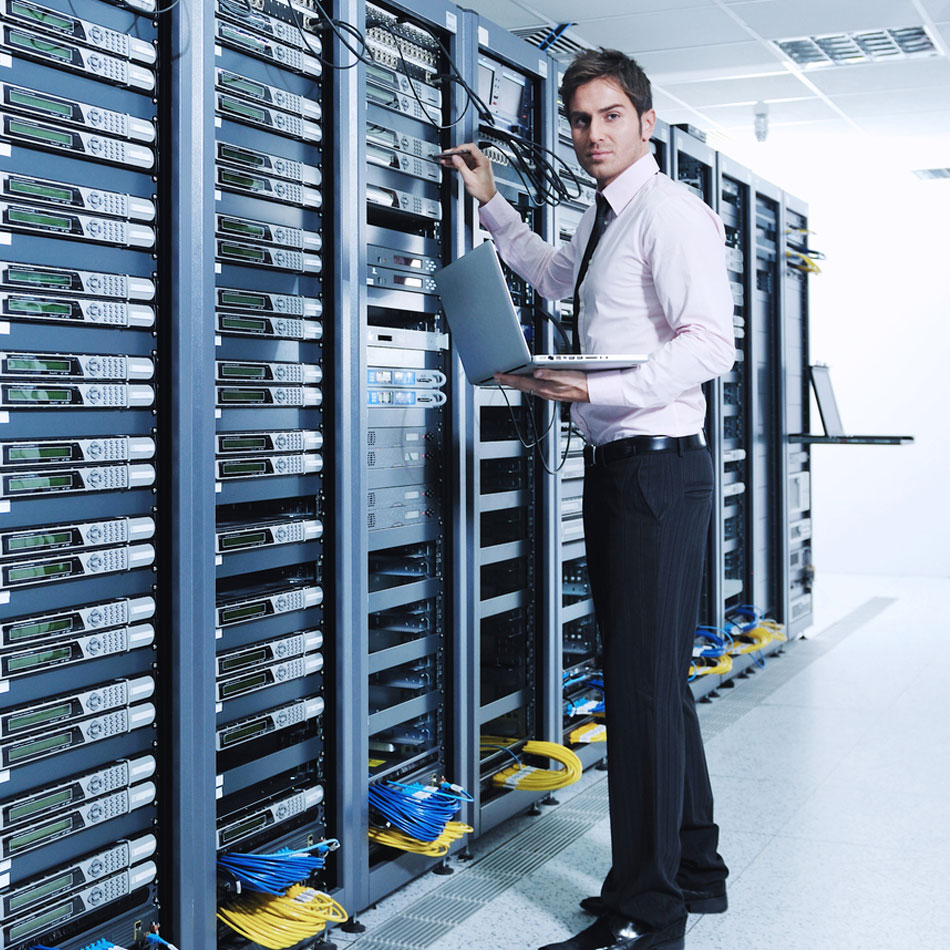
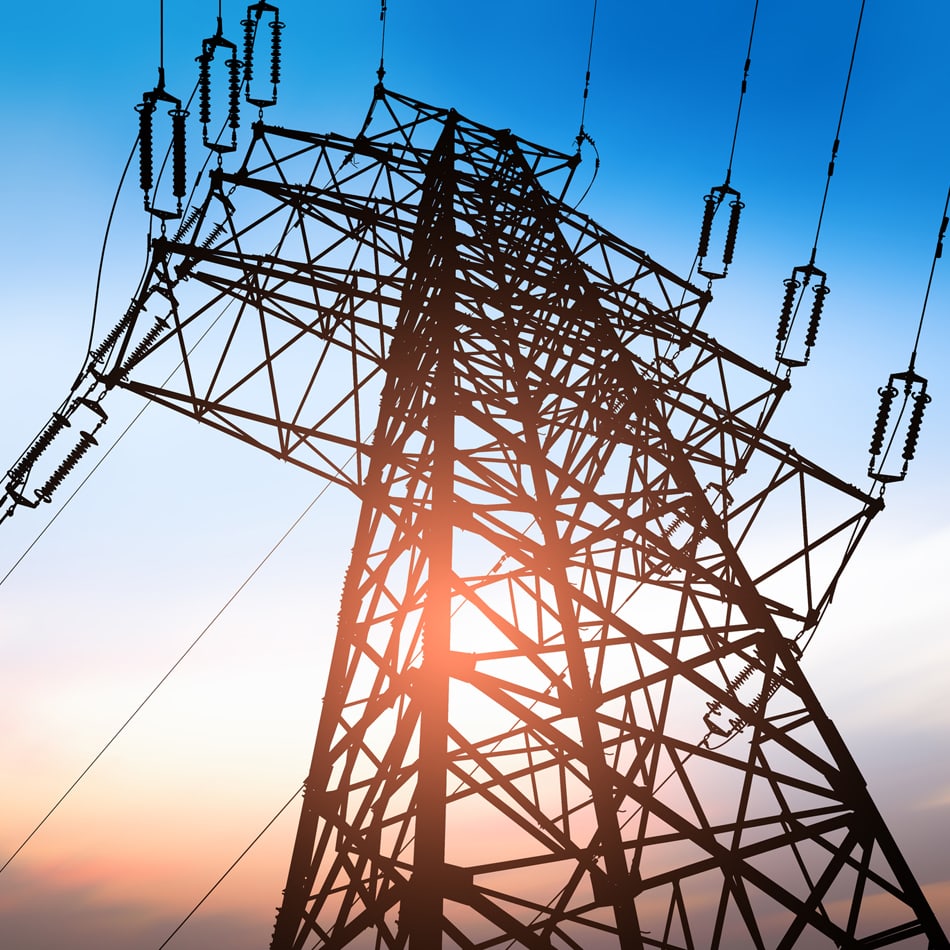
The Georgia Tech Global Learning Center and Georgia Tech-Savannah campus is compliant under the Americans with Disabilities Act. Any individual who requires accommodation for participation in any course offered by GTPE should contact us prior to the start of the course.
Courses that are part of certificate programs include a required assessment. Passing criteria is determined by the instructor and is provided to learners at the start of the course.
CEUs are awarded to participants who attend a minimum of 80% of the scheduled class time.
Georgia Tech’s Office of Research Security and Compliance requires citizenship information be maintained for those participating in most GTPE courses. Citizenship information is obtained directly from the learner at the time of registration and is maintained in the Georgia Tech Student System.
Learners enrolled in any of Georgia Tech Professional Education's programs are considered members of the Georgia Tech community and are expected to comply with all policies and procedures put forth by the Institute, including the Student Code of Conduct and Academic Honor Code.
Please refer to our Terms and Conditions for complete details on the policies for course changes and cancellations.
Participants in GTPE courses are required to complete an online profile that meets the requirements of Georgia Tech Research Security. Information collected is maintained in the Georgia Tech Student System. The following data elements are considered directory information and are collected from each participant as part of the registration and profile setup process:
- Full legal name
- Email address
- Shipping address
- Company name
This data is not published in Georgia Tech’s online directory system and therefore is not currently available to the general public. Learner information is used only as described in our Privacy Policy. GTPE data is not sold or provided to external entities.
Sensitive Data
The following data elements, if in the Georgia Tech Student Systems, are considered sensitive information and are only available to Georgia Tech employees with a business need-to-know:
- Georgia Tech ID
- Date of birth
- Citizenship
- Gender
- Ethnicity
- Religious preferences
- Social security numbers
- Registration information
- Class schedules
- Attendance records
- Academic history
At any time, you can remove your consent to marketing emails as well as request to delete your personal data. Visit our GTPE EU GDPR page for more information.
Classes and events being held at the Georgia Tech Global Learning Center in Atlanta or Georgia Tech-Savannah campus may be impacted by closures or delays due to inclement weather.
The Georgia Tech Global Learning Center will follow the guidelines of Georgia Tech main campus in Atlanta. Students, guests, and instructors should check the Georgia Tech homepage for information regarding university closings or delayed openings due to inclement weather. Please be advised that if campus is closed for any reasons, all classroom courses are also canceled.
Students, guests, and instructors attending classes and events at Georgia Tech-Savannah should check the Georgia Tech-Savannah homepage for information regarding closings or delayed openings due to inclement weather.
GTPE certificates of program completion consist of a prescribed number of required and elective courses offered and completed at Georgia Tech within a consecutive six-year period. Exceptions, such as requests for substitutions or credit for prior education, can be requested through the petition form. Exceptions cannot be guaranteed.
Please refer to our Terms and Conditions for complete details on the policy for refunds.
Georgia Tech is a tobacco-free and smoke-free campus. The use of cigarettes, cigars, pipes, all forms of smokeless tobacco, and any other smoking devices that use tobacco are strictly prohibited. There are no designated smoking areas on campus.
Courses that are eligible for special discounts will be noted accordingly on the course page. Only one coupon code can be entered during the checkout process and cannot be redeemed after checkout is complete. If you have already registered and forgot to use your coupon code, you can request an eligible refund. GTPE will cancel any transaction where a coupon was misused or ineligible. If you are unsure if you can use your coupon code, please check with the course administrator.
GTPE does not have a program for senior citizens. However, Georgia Tech offers a 62 or Older Program for Georgia residents who are 62 or older and are interested in taking for credit courses. This program does not pay for noncredit professional education courses. Visit the Georgia Tech Undergraduate Admissions page for more information on the undergraduate program and Georgia Tech Graduate Admissions page for more information on the graduate program.
There is no special process or form to register your group. All interested learners must create and manage their own individual profiles, accounts, and registrations.
- Complete a GTPE profile.
- Shop for a course.
- Add the course(s) to the cart.*
- Apply a group discount code (if applicable).
- Provide an accepted payment method to complete the order (credit card, third party credit card holder, or one accepted payment document).
*Carts will remain active for 14 days, but seats are not held until the transaction is complete.
Courses that offer group discounts will display the discount code on the course page. Your employees will use the code during the registration process and cart totals will adjust accordingly. Group discounts can only be used if three or more employees from the company attend the same course and only one coupon code can be use per shopping cart.
If you have already registered and forgot to use your coupon code, you can request an eligible refund.
Accepted payment documents must be uploaded during the registration process. They include:
- A company purchase order (PO or SF182)
- A letter of authorization on company letterhead
- A corporate education application/voucher
- Name of company and physical address
- Name of employee(s) approved for training
- Document number (SF-182 documents: Section C, Box 4)
- Billing address (SF-182 documents: Section C, Box 6)
- Course title and course dates
- Maximum disbursement amount (billing amount)
- Expiration date (if applicable)
- Authorized signature(s)
- Payment terms less than or equal to net 30
The employee can print of a copy of their shopping cart to submit if required for payment documents. The cart will remain active for 14 days, but the seat will not held until registration and payment is complete.
Registrations cannot be processed without payment. If your employee is concerned about losing a seat in a class because of internal company processes, we suggest that they go ahead and register and pay with a personal or corporate credit card and seek reimbursement.
If you need assistance with your group registration or have questions on how to start the process, please feel free to contact us at 404-385-3501 or peregistration@gatech.edu.
Most GTPE classroom courses are held at the Georgia Tech Global Learning Center (GLC). Any courses that are held elsewhere will be clearly marked on the course page. Get information on parking, directions, and transportation to the GLC.
We do not provide overnight rooms. However, accommodations can be made at the Georgia Tech Hotel and Conference Center, adjacent to us. Additional hotels can be found within walking distance. Get more information on accommodations.
Learners may transfer to another course of equal or greater cost if notification is made at least 10 business days prior to the original course start date. The course to which one transfers must already be scheduled.
We recommend you register for courses as early as possible. Session details will indicate when there is less than five reamining seats in a particular session.
Updates to your company, address, email, phone, and passwords can be made directly on the GTPE website. Name changes and citizenship changes must be submitted to the GTPE Registrar’s Office.
- Complete a GTPE profile.
- Shop for a course.
- Add the course(s) to the cart.*
- Apply a special discount code (if applicable).
- Provide an accepted payment method to complete the order (credit card, third party credit card holder, or one accepted payment document).
*Carts will remain active for 14 days, but seats are not held until the transaction is complete.
Walk-in registrations are accepted based on space availability but are not guaranteed for any courses.
If available, discounts will display on the course page or will be automatically applied during the purchase process. Only one coupon code should be entered during the checkout process and will be validated by the system if applicable to items in your cart. If you have already registered and forgot to use your coupon code, you can request an eligible refund.
GTPE does not have a discount program for senior citizens. However, Georgia Tech offers a 62 or Older Program for Georgia residents who are 62 or older and are interested in taking for credit courses. This program does not pay for noncredit professional education courses. Visit the Georgia Tech Undergraduate Admissions page for more information on the undergraduate program and the Georgia Tech Graduate Admissions page for more information on the graduate program.
GTPE does not have a program for senior citizens. However, Georgia Tech offers a 62 or Older Program for Georgia residents who are 62 or older and are interested in taking for credit courses. This program does not pay for noncredit professional education courses. Visit the Georgia Tech Undergraduate Admissions page for more information on the undergraduate program and the Georgia Tech Graduate Admissions page for more information on the graduate program.
In the event of a cancellation, we will provide you with a full refund or transfer to an equivalent course.
Short courses (1-5 days) and conferences do not require a student visa. A B-2 Tourist Visa, along with a copy of your registration confirmation email and a copy of your completed web registration order page, should suffice.
If participation in a course is employment related, with immediate departure from the U.S., then a B-1 Temporary Business Visa will be required.
We encourage you to contact your U.S. Consulate or Embassy to determine visa eligibility. Full refunds will be provided to participants who are unable to obtain an entry visa and contact our office prior to the start of the course.
English as a Second Language students should contact the Language Institute for admission and visa requirements.
We do not issue letters of invitation and cannot provide immigration documents for the issuance of a student visa. Full refunds will be provided to participants who are unable to obtain an entry visa and contact our office prior to the start of the course.
If you need assistance with your group registration or have questions on how to start the process, please feel free to contact us at 404-385-3501 or peregistration@gatech.edu.
Full payment is due at time of registration. Accepted payment methods include:
- Credit cards
- Purchase orders (company and government)
- International wire payments*
- Georgia Tech Workday Number (for Georgia Tech employees only)
- Private loans*
- Company checks*
* Requires document upload or transaction verification during the checkout process.
Purchase order documents must include the following:
- Name of company and physical address
- Name of company’s financial contact and/or ap (accounts payable) email address
- Name of employee(s) approved for training
- Document number (SF-182 documents: Section C, Box 4)
- Billing address (SF-182 documents: Section C, Box 6)
- Course title and course dates
- Maximum disbursement amount (billing amount)
- Expiration date (if applicable)
- Authorized signature(s)
- Payment terms less than or equal to net 30
Please do not include social security numbers on purchase order documents.
- Make your check payable to “Georgia Institute of Technology” and include the order number and participant name on the face of the check.
- Choose “Company Purchase Order” as the payment method at checkout and upload a copy of your check to your order.
- Mail your check to:
GTPE Accounting
Georgia Institute of Technology
Global Learning Center
84 5th St. NW
Atlanta, GA 30308-1031
Full payment is due at the time of registration.
General Public
Payment is due at the time of purchase. Invoice payments must adhere to the Board of Regent’s business terms of net 30.
Georgia Tech Employees
PeopleSoft payments are processed at the time of registration. Georgia Tech employees cannot use PCards for GTPE registration charges.
Additional fees vary by course. Be sure to review the Requirements & Materials tab on the course page for more information.
Yes. Here are the steps to receive an invoice:
- Complete a GTPE profile.
- Shop for a course.
- Add the course(s) to the cart.
- Print your cart and submit to your employer as the cost estimate.
- Receive a copy of your company’s Purchase Order or payment approval document for GTPE to invoice against.
- Return to your cart, proceed through checkout and upload our company PO in the final payment step.
- The GTPE Business Office will generate an invoice 30 days prior to the start of the course at which point you are no longer eligible to withdraw with refund.
Your company must:
- Abide by the Georgia Tech and Board of Regent’s business terms of net 30.
- Pay the full balance of a Georgia Tech invoice (there are no discounts for payments made early or on time).
- Pay the invoice if the employee fails to withdraw during the refund period and does not attend the course.
Credit card refunds are processed to the original credit card. The credit card issuer is responsible for refund credit balances to the cardholder.
We do not offer payment plans for any of our services, conferences, or courses. Payment must be made in full at time of purchase.
GTPE cannot issue 1098-T tax forms. If you have a payment history need for tax purposes, we are happy to provide you with receipts of payment. Please submit your requires to peregistration@gatech.edu. Be sure to include your full legal name and Georgia Tech ID which can be found within your GTPE profile.
Please see our Terms and Conditions for complete details on our policies for course registration changes.
GTPE’s use of CEU follows accepted criteria and guidelines established by the Georgia Board of Regents which follows international standards such as The International Association for Continuing Education and Training (IACET).
GTPE does not issue transcripts or certificates with Professional Develop Hours (PDH) or Professional Development Units (PDU), but the crosswalk here is provided for reference.
One CEU = 10 contact hours of instruction
One PDH = 1 contact hour of instruction (one CEU = 10 PDH)
One PDU = 1 contact hour of instruction (one CEU = 10 PDU)
Upon successful completion of most GTPE courses (80% minimum attendance and a passing grade in courses that require an assessment), you may receive a certificate indicating the number of CEUs earned. Certificate issuance exceptions include courses with outstanding credentialing entities (i.e. OSHA or PADI).
CEUs earned are recorded in the attendee’s name and will appear on a GTPE transcript. All transcripts must be requested by the attendee via the transcript request form. Requests are typically processed within three business days.
For an audit of your transcript for progress toward completion of a certificate, please complete the transcript request form. GTPE courses do not provide academic or degree credit. Georgia Tech academic or degree credit is only available to matriculated students taking courses that meet degree requirements.
For specific information on state licensing or credit requirements, please contact your state licensing board. If you are seeking certification through a professional association, please review the specific requirements with that association.
TRAIN AT YOUR LOCATION
We enable employers to provide specialized, on-location training on their own timetables. Our world-renowned experts can create unique content that meets your employees' specific needs. We also have the ability to deliver courses via web conferencing or on-demand online videos. For 15 or more students, it is more cost-effective for us to come to you.
-
Save Money
-
Flexible Schedule
-
Group Training
-
Customize Content
-
On-Site Training
-
Earn a Certificate